Poisson Flow Generative Models
TOC
Reference:
Official links:
-
PFGM (NeurIPS 2022)
Blog:
Main idea
The idea is inspired by the field of electrodynamics that Any distribution of electrons in a hyperplane generates an electric field (aka Poisson field) that transforms the distribution into a uniform angular distribution as the distribution evolves through time according to the dynamics defines by the field.
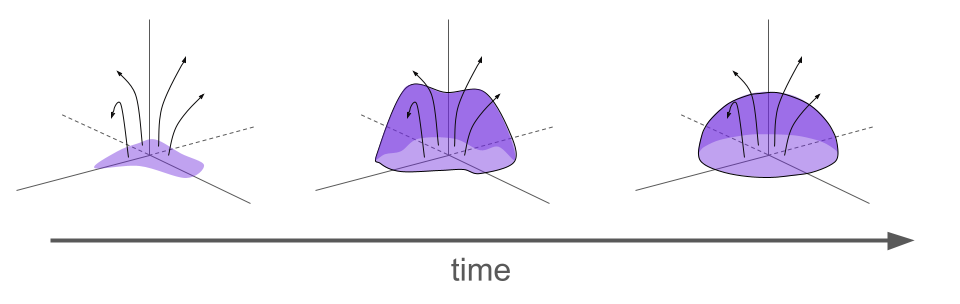
If we know the electric field (Poisson field) generated by a distribution, then we can start with points uniformly sampled on a hemisphere and run the dynamics in reverse time to recover the original data distribution. The law of physics, therefore, provide an invertible mapping between a simple distribution and the data distribution, yielding a means to generate novel data akin to normalizing flows.

Training process
We seek to model the dynamics of particles under the influence of the Poisson field generated by a data distribution.
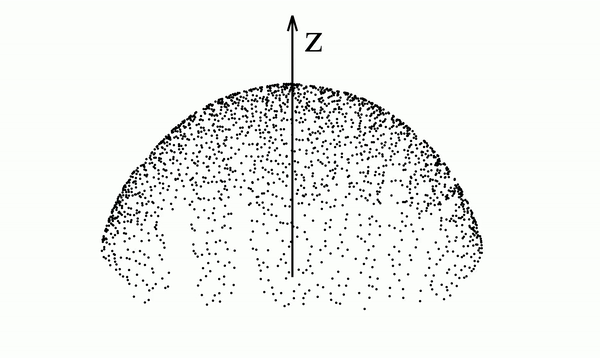